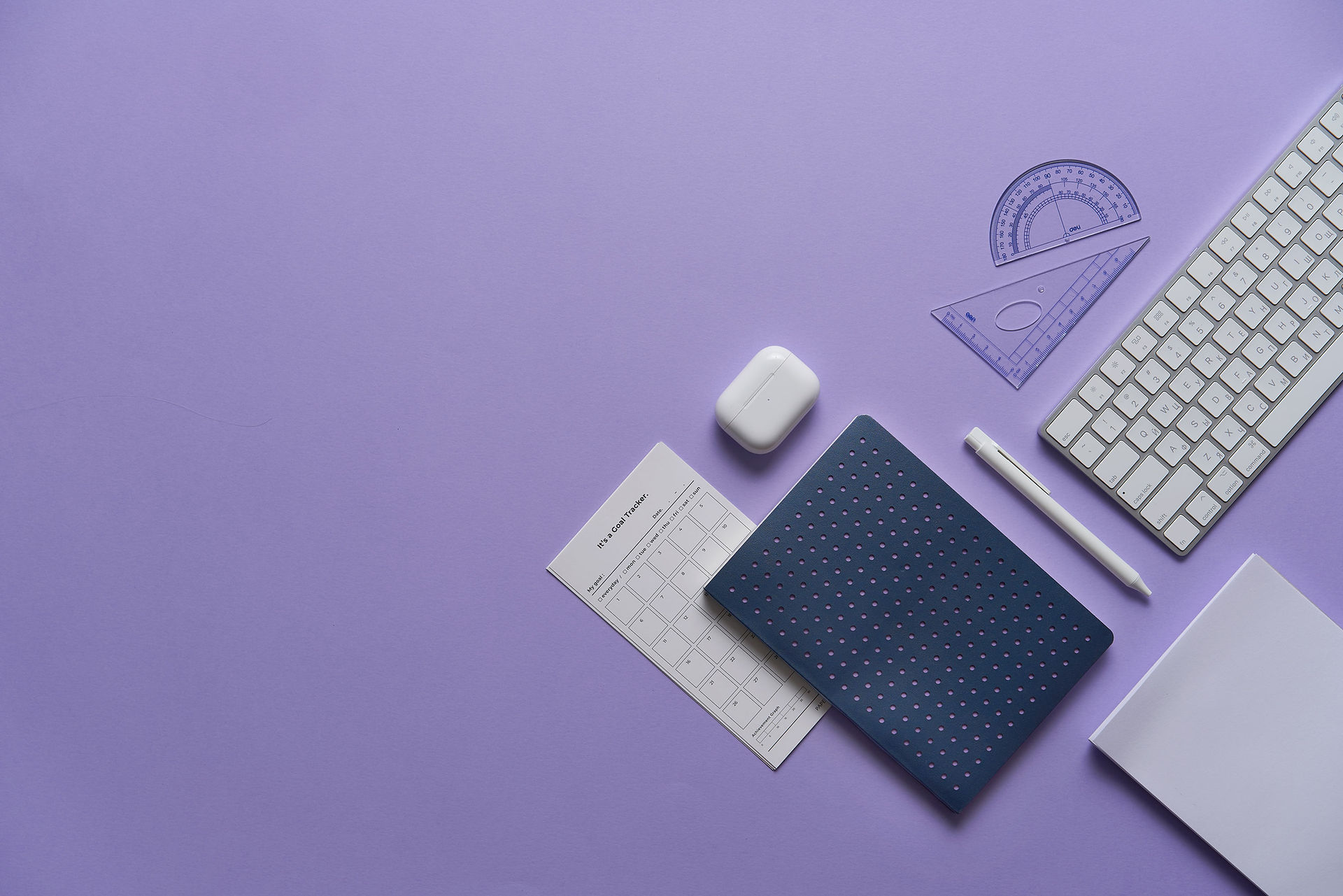
What Aspect Do You Like: Multi-scale Time-aware User Interest Modeling for Micro-video Recommendation
Abstract
Online micro-video recommender systems aim to address the information explosion of micro-videos and make personalized recommendations for users. However, the existing methods still have some limitations in learning representative user interests, since the multi-scale time effects, user interest group modeling and false positive interactions are not taken into consideration. In view of this, we propose an end-to-end multi-scale time-aware user interest modeling network (MTIN). We first present an adaptive interest group routing algorithm to generate fine-grained user interest groups, meanwhile, a discount factor is introduced to reduce the detrimental effects of false-positive interactions. Afterwards, to explore multi-scale time effects on user interests, an item-level and group-level interest extraction unit is designed, and then we distill the user representation from fine-grained interest groups. At last, the user representations and micro-video candidates are fed into a deep neural network (DNN) for predictions. Extensive experiments on two real-world datasets demonstrate that MTIN achieves substantial gains over the state-of-the-art methods.